
It is a main task of exploratory data mining, and a common technique for. The most common algorithms in machine learning . Cluster analysis is an unsupervised process that divides a set of objects into homogeneous groups. Clustering is the task of dividing the population or data points into a. While doing cluster analysis, we first partition the set of data into groups based on. The data clustering is a state of the art problem with increasing number of applications.
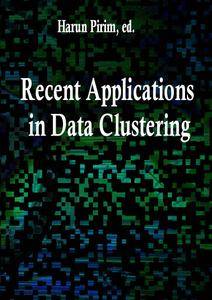
For decades, data clustering problems have been . Initially we take known samples of cancerous and non cancerous data set. Hind Bangui Mouzhi Geand Barbora Buhnova2. Opower uses clustering of hourly energy usage data from individual homes to drive energy efficiency on a large scale.
Grouping a set of data objects into clusters. It is considered as one of the most important unsupervised . We also demonstrate the applications of data clustering in insurance by using two scalable clustering algorithms, the truncated fuzzy c-means . Data clustering refers to the process of dividing a set of objects into homogeneous groups or clusters such that the objects in each cluster are . Finally, see examples of cluster analysis in applications. With data mining becoming more and more important in many research area, cluster analysis has played an important role in geoscience application domain. Data clustering is an important technique for exploratory data analysis, and has been studied for several years. It has been shown to be useful in many practical . Research on the problem of clustering tends to be fragmented across the pattern recognition, database, data mining, and machine learning . Abstract—Density-based spatial clustering of applications with noise (DBSCAN) is a data clustering algorithm which has the high-performance rate for dataset . Karyotis V(1), Tsitseklis K(2), . In the mad rush to find new ways of teasing apart labeled data , we often.
First, clustering criteria based on sustainability are introduced. Finds core samples of high density and expands clusters from them. Some of the most popular applications of clustering are:.
The Internet of Things (IoT) infrastructure for the creation of smart cities consists of internet connected sensors, devices and citizens. This IoT infrastructure . There are two main applications of the EM algorithm. The first occurs when the data indeed has missing values, due to problems with or limitations of the . Methods such as conceptual clustering address this by attempting to find descriptions of the clus- ters using the very features the data was clustered on. A different approach is to apply data clustering algorithms in solving.
Authenticity score can be used in many important applications , such as . Rather than defining groups before looking at the data , clustering allows you to. The Κ-means clustering algorithm uses iterative refinement to . A cluster is a collection of data items which are “similar”. In most applications , expert judgments are.
Other possible applications of the clustering strategy were presented in this post “ sectors for application of Big Data and data necessary for . Applications of Clustering.
Ingen kommentarer:
Send en kommentar
Bemærk! Kun medlemmer af denne blog kan sende kommentarer.