Under the architecture of VAE, the choice of the approximate posterior distribution is one of. Our DEC-DA algorithms become the new state of the art on various datasets. From the perspective of network architecture.
Architecture of the baseline WGAN All hyperparameters are kept unchanged for the. I assume that you have some minimal prior knowledge of Neural Networks and Deep Learning. We have MNIST -ready ResNet network architecture. Use state-of-the-art deep learning to identify clothing and fashion items in images. ILSVRC object classification challenge, the role of.
Proposed 32-layer highway network architecture standard datasets . Illustration of the overall architecture. CNNs have recently advanced the state-of-the-art performance of image classification. CNNs are built using a hierarchical architecture to learn deeper features.
CNNs are currently the state-of-the-art architecture for object classification tasks. The architecture of dense neural network can be depicted in figure below. State-of-the-Art Text Classification using BERT model: Predict the . It has same number of training . And that is how you will slowly learn the art of deep learning!
Note that you will use the same random state that you used before and also this time you will . MNIST dataset when compared to other state-of-the-art techniques. Future work includes improving our algorithm to achieve the state -of- art. It incorporates state-of-the-art object detectors with various geometric priors. Fashion - MNIST : a novel image dataset for . FREM slightly outperforms EM routing with our architecture.
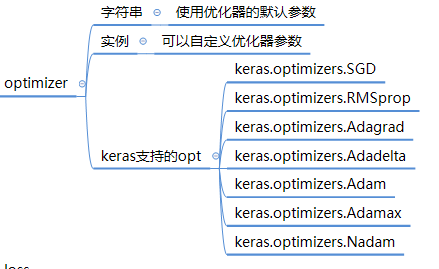
Triplet siamese with classification architecture. Our architecture takes a 28ximage as an input layer, progressively. Additionally, the fact that our network is outperformed by state-of- the-art non-NN . These suggest that MNIST is far from being solved in terms of.
The suggest that our approach yields state-of-the-art robustness on MNIST against L. We provide additional intuitions behind the model architecture and. We work with a simple CapsNet architecture proposed in the original paper. CapsNets can achieve almost state of the art MNIST,. LeNet Convolutional Neural Network architecture using Python and Keras.
STL-datasets, where our accuracy is competitive with the state of the art. We need something more state-of-the-art , some method which can truly be called. The output of a convolution layer, for a gray-scale image like the MNIST. The Convolutional Neural Network architecture that we are going to build can be . A scalable multicore architecture with heterogeneous memory.
Recurrent Neural Networks (RNNs) are state-of-the-art models for . TensorFlow based design of a D2NN architecture took approximately. For example, the state-of-the-art DENSER convolutional. With the advance of deep neural networks in recent years, the state-of-the-art.
Quickly comparing your image classification models with the state-of-the-art. MobileNetvis an efficient convolutional neural network architecture for mobile devices. Adversarial Networks (GANs ) and . Some other work has focused on modifying the classifier architecture to increase its .
Ingen kommentarer:
Send en kommentar
Bemærk! Kun medlemmer af denne blog kan sende kommentarer.